Lowkey-Advanced Ridge Regression (Part I)
I Studied Regressions *Only* for 30 Days So You Don't Have To But You Have To Subscribe
In case some of you are not fully invested in my life yet, actually I started a self challenge where I study regressions only for 30 days:
Day 11 out of 30 of studying regressions *only*
— quantymacro (@quantymacro) March 12, 2024
(yes I’m in that phase of going through old materials in hope to find some novel insights and pass them off as my own) pic.twitter.com/XjBTTkmSes
So of course what good would it be if I had spent 30 days studying a technique and not write an article to shill the technique I read about? You're welcome.
Last time we went in depth into LASSO and put it to the test. We noted that LASSO can recover sparsity well, but only in limited circumstances. And given the lack of invariance of regularisation under change of basis, I thought that dropping variables seems a bit extreme, given that the basis of the predictors are arbitrary. Shrinking them seems a bit more permissible. (I removed the paywall btw so feel free to read it)
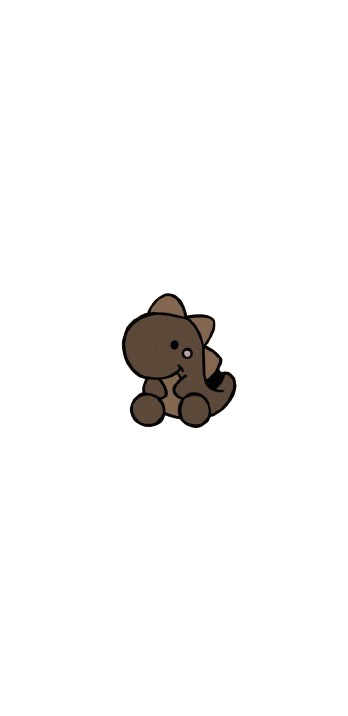
We gave some handwavy explanation on why Ridge is a sensible alternative, but we never really went deep into it. In this article we will try to sharpen our intuition and understanding of Ridge Regression. Specifically we will try to touch on these topics:
What are the exact conditions where Ridge regularization will add value?
Effects of dense true coefficients on optimal Ridge penalty
Tips on finding Ridge penalty in cross-validation